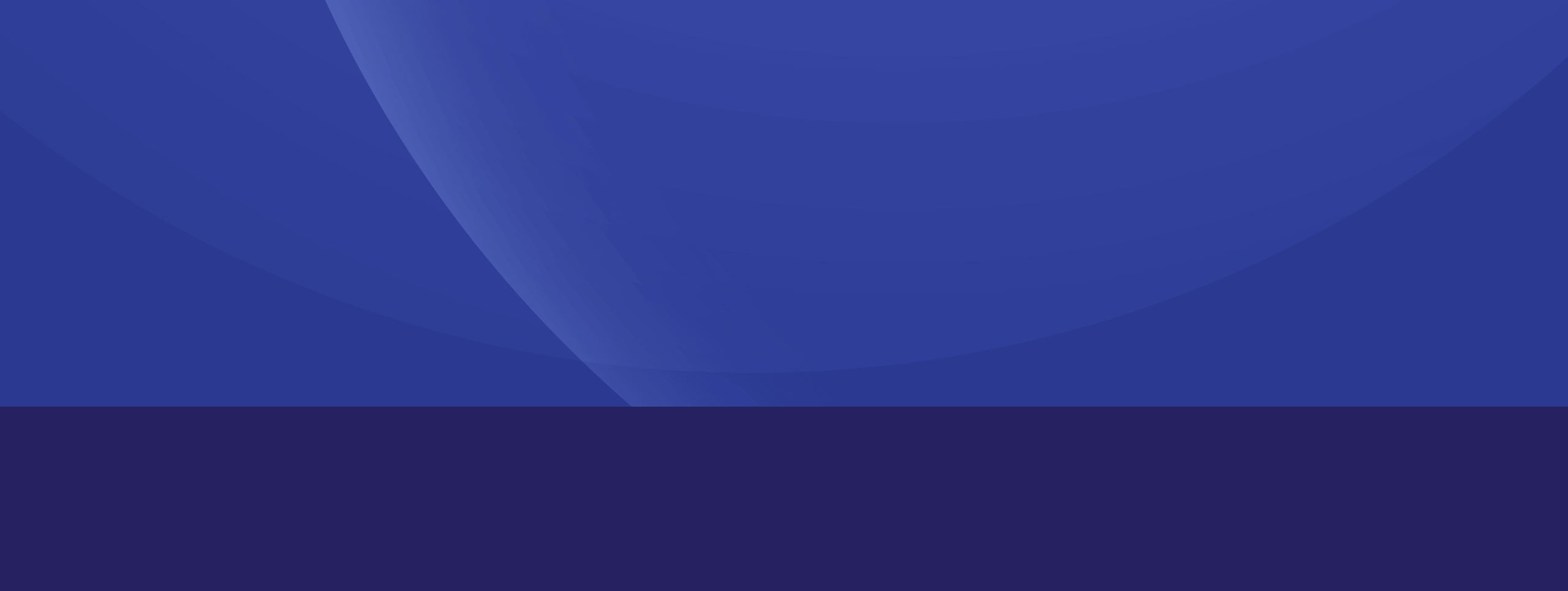
Innovation
UNOS researchers discover a new way to predict kidney utilization rates
Using natural language processing (NLP) to predict which kidneys will be utilized may improve organ acceptance rates.
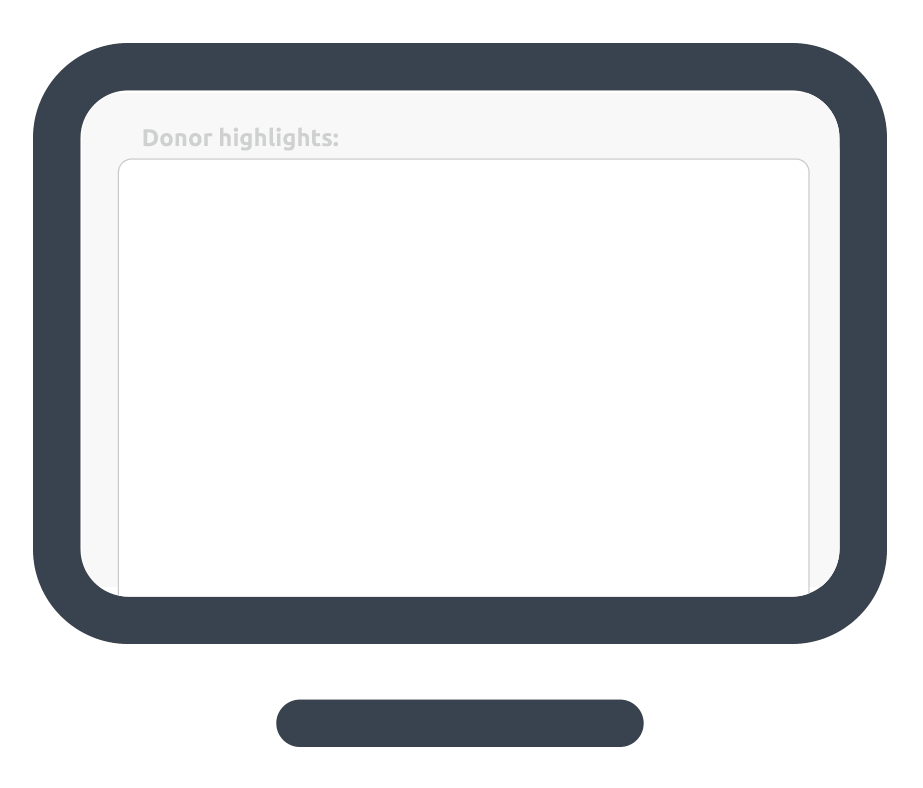
Researchers at United Network for Organ Sharing have used natural language processing (NLP) to predict which deceased donor kidneys will experience placement difficulties and to forecast which will be accepted by transplant hospitals. The innovative predictive methodology has the potential to enhance existing models, paving the way for improved organ utilization rates at organ transplant hospitals across the country.

“Often the only way to improve model performance is by incorporating new data sources or finding new insights within the data.”
Andrew Placona, UNOS data science manager
“Our analysis demonstrates that free text donor narratives could be a potent, untapped data source that could augment our current prediction of kidney utilization,” said UNOS data science manager Andrew Placona. Placona is corresponding author in a new proof-of-concept article published in the American Journal of Transplantation that explores the possibility of using NLP to mine the free text fields of deceased donor registration forms for information that might predict kidney utilization rates. Existing predictive algorithms have only leveraged structured data, such as yes or no questions, which may not provide sufficient detail to enable fully informed organ offer acceptance.
Opportunities for improvement with NLP
Approximately 13 end-stage renal disease patients die each day awaiting a kidney transplant in the U.S., according to data from the Organ Procurement and Transplantation Network, while 20 percent of kidneys recovered from deceased donors are not transplanted. “The potential to improve existing kidney utilization prediction models is limited if further attempts are based solely on the same traditional OPTN data sources,” Placona said. “Often the only way to improve model performance is by incorporating new data sources or finding new insights within the data.”
The UNOS research team, which included the national nonprofit’s director of research and chief medical officer, proposed that natural language processing could be used to analyze large sets of nuanced clinical text that may be associated with kidney utilization rates. A subfield of linguistics, computer science and artificial intelligence, NLP uses computers to process and analyze large amounts of natural language data to gain otherwise imperceptible insights. “We’re using data that traditionally UNOS has never touched,” Placona said. Placona’s team performed the research through UNOS LabsSM, which is an experimental incubator that brings together data, technology and industry expertise to improve the transplant system and increase the number of organ transplants performed. “No one has really looked at the clinical text yet and it’s gone unnoticed for decades because it is not easy data to work with,” he said.
Predicting organ placement difficulties
In the study, the researchers developed a natural language processing-based model to predict the probability of organ preservation for nearly 20,000 adult deceased kidney donors between 2010 and 2018, leveraging free text from the deceased donor registration forms that are completed by organ procurement coordinators as part of the donor assessment process. The unstructured data came from three free text fields—admission course history, donor medical and social history and donor highlights.
The natural language processing text-based prediction model was moderately correlated to existing models that predict deceased donor kidneys that will experience placement difficulties, but it offered novel insights. “The free text complements all the other data and yields a similar level of predictive power as traditional indices developed using structured data,” Placona said. “Knowing our findings align with existing literature is encouraging when evaluating some of the more novel factors identified by the natural language processing model. It offers some promise to potentially improve what is out there.”
Free text fields could contain specific reasons for increased risk of infection with HIV, hepatitis B or hepatitis C, which may not be captured elsewhere in the deceased donor registration form. The forms could also describe how a person became a deceased organ donor, which wouldn’t be captured through structured data. In the journal article, Placona provided the following illustrative example of text that might be included in the admission course field: “Patient was found in room with needles nearby. Downtime unknown. Naloxone administered on scene.”
Novel key social factor terms predicting transplant included those that indicated recreational, non-intravenous drug usage such as the words beer and marijuana, as well as words that indicated that the donor was young with an active support network, such as the words mother and student. “Some intuitive patterns emerged from the word lists,” Placona said.
Encouraged by the study findings, the UNOS team plans to conduct more research to better understand the potential to enhance existing predictive models. “Although we have demonstrated that natural language processing methods on free text donor narratives hold promise, we recognize that more work needs to be done,” Placona said. “With the right analytical approaches, combined with more disparate data sources, we can further refine our existing utilization models and leverage techniques to understand what is driving the predictions.”
Placona, A., Martinez, C., McGehee, H., Carrico, B., Klassen, D.K. and Stewart, D. (2019), Can Donor Narratives Yield Insights? A Natural Language Processing Proof of Concept to Facilitate Kidney Allocation. Am J Transplant. Accepted Author Manuscript. doi:10.1111/ajt.15705
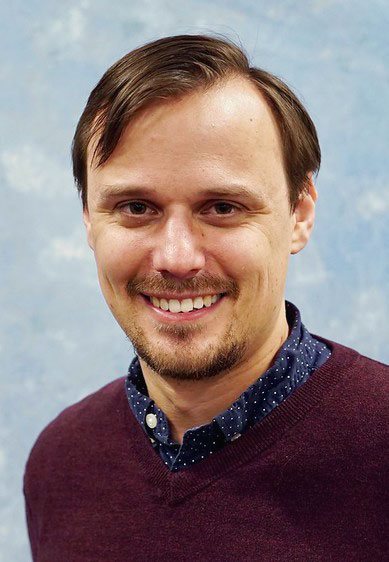
Placona joined UNOS as the nonprofit’s first data science manager in May 2018. Prior to joining UNOS, he worked as a data analyst in the financial and health care industries, where he applied natural language processing to drive business insights. Placona has bachelor’s degrees in history and music from Roanoke College, as well as a master’s degree in public policy analysis from Carnegie Mellon University. He is currently pursuing a doctorate in health services organization and research at Virginia Commonwealth University.