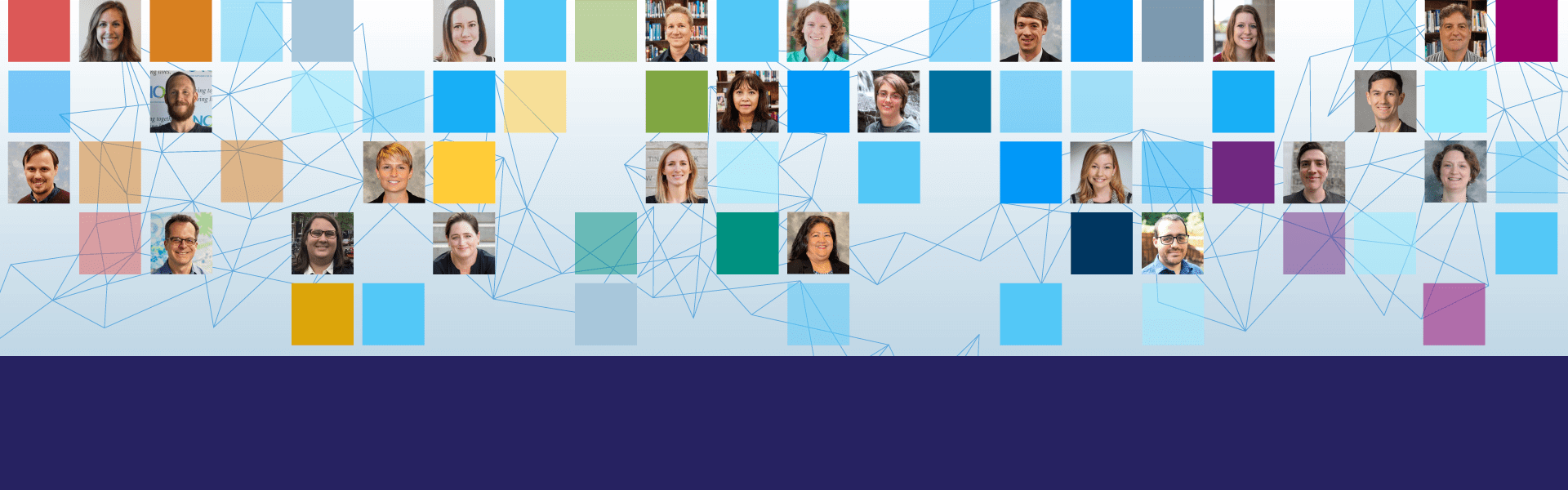
UNOS researchers apply machine learning techniques to OPTN data.
in focus
Age and body mass index were among the top features to appear in the machine learning predictive model.
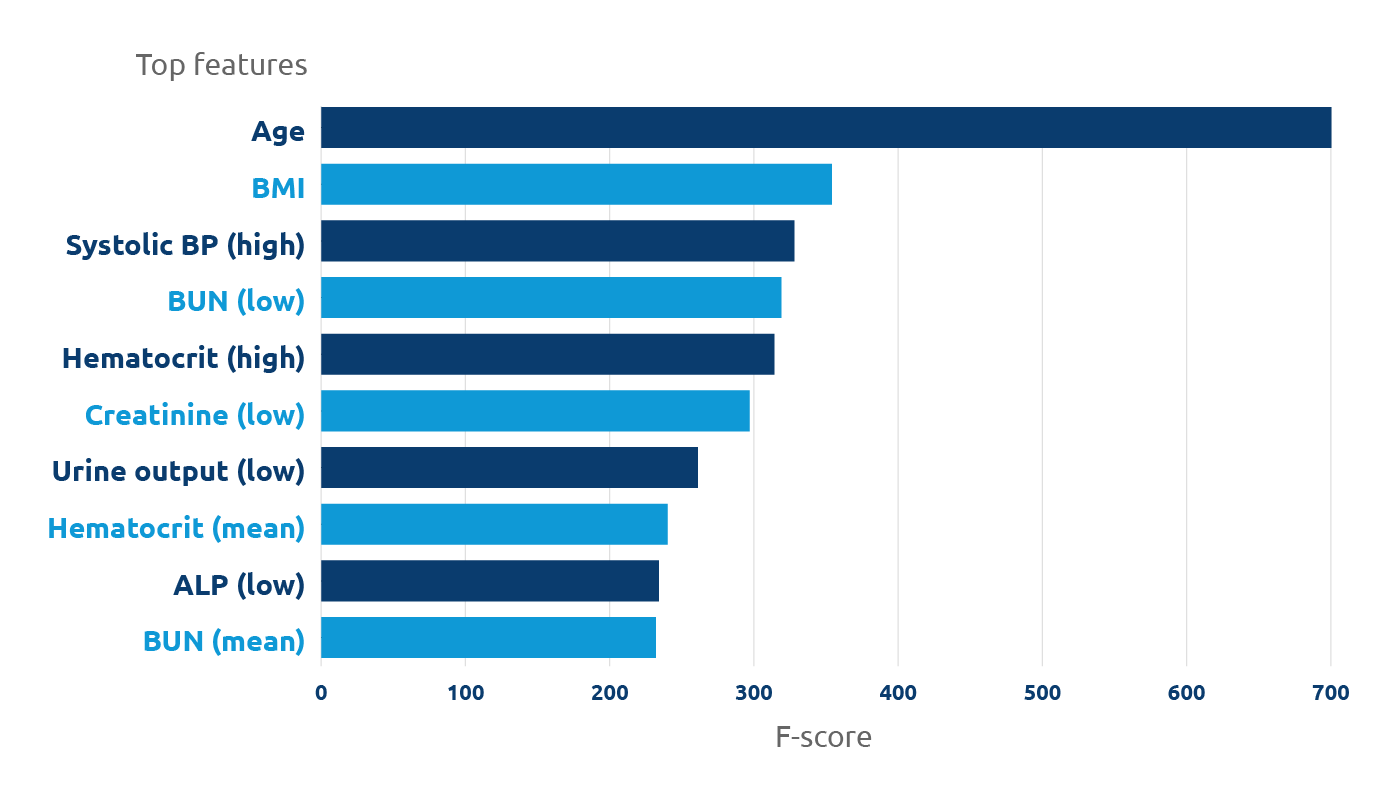
Biopsy findings are often cited by transplant teams as a key factor in determining whether to accept a deceased kidney offer, despite inconclusive evidence that kidney biopsy findings are associated with graft outcomes. In an effort to address the discrepancy and improve kidney allocation rates, two United Network for Organ Sharing researchers conducted a study using machine learning to predict deceased donor kidney biopsy results.
“We were motivated to do the research based on the influential role of biopsy findings on kidney allocation,” said UNOS associate data scientist Carlos Martinez, who conducted the research along with data science manager Andrew Placona. “We wanted to determine if biopsies served as representations of other clinical data.”
The pair were among more than two dozen UNOS researchers who presented their data-driven studies aimed at discovering innovations that improve the U.S. organ donation and transplantation system at the 2020 American Transplant Congress.
Predictive performance
Martinez and Placona developed and evaluated a machine learning model to predict biopsy results using only the clinical and lab data that was available in DonorNet® at the time of the match.
In examining Organ Procurement and Transplantation Network data for approximately 33,000 deceased donors since 1999, they sought to predict the severity of kidney glomerulosclerosis, or GS. To do so, they identified variables that could be associated with kidney biopsy findings, such as age, ethnicity, history of hypertension and serum creatinine levels. They then mapped the clinical and lab data to five categories of GS severity using the machine learning predictive model.
“We observed markedly different performance across categories,” Martinez said, adding that age and body mass index were among the top features to appear in the predictive model. The model had better predictive performance for the extreme categories of very low or very high GS relative to the performance for intermediate GS. The researchers suggest this could be due to a phenomenon known as restricted variance, in which the variability of donor characteristics is greater for the intermediate GS categories than the extreme categories. The increased variability could hinder predictive performance in the intermediate categories.
Based on the study results, however, the researchers foresee challenges for future automated biopsy prediction models.
“A key challenge is that the reliability between pathologists may vary depending on the level of GS,” Martinez said, adding that there may be a lack of agreement among pathologists from a single biopsy. They concluded that further investigation is needed to assess confidence of biopsy results stratified by severity and the impact on predictive models derived from those results.
“The results highlight that predicting biopsy results based on clinical and lab data is challenging, but the difference in performance across GS categories may inform areas where the model can be improved,” Martinez said.
Martinez C, Placona A. Machine Learning to Predict Deceased Donor Kidney Biopsy Results [abstract]. Am J Transplant. 2020; 20 (suppl 3).
Carlos Martinez, UNOS associate data scientist
Read more
In focus
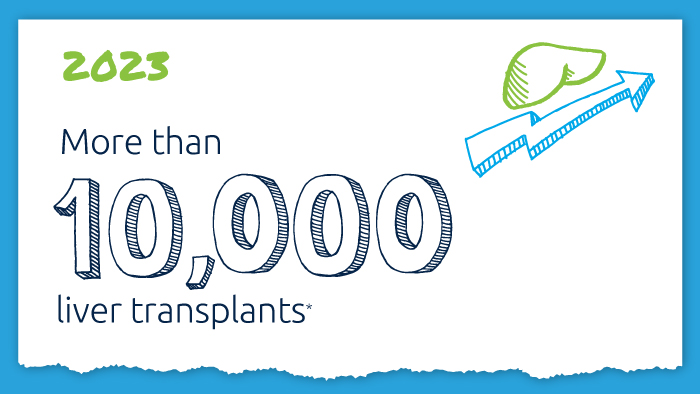
A decade of record increases in liver transplant
10,660 liver transplants, the most ever in a year.
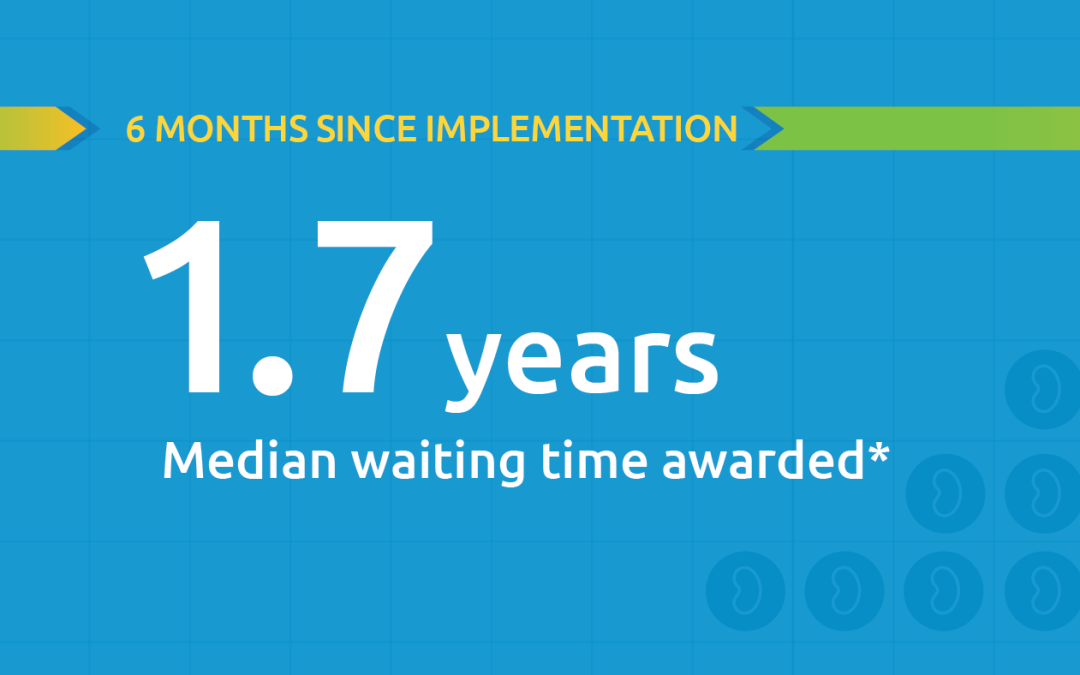
Black kidney candidates are receiving waiting time modifications, helping them get the organs they need
Latest kidney monitoring report shows two new kidney polices are working as intended
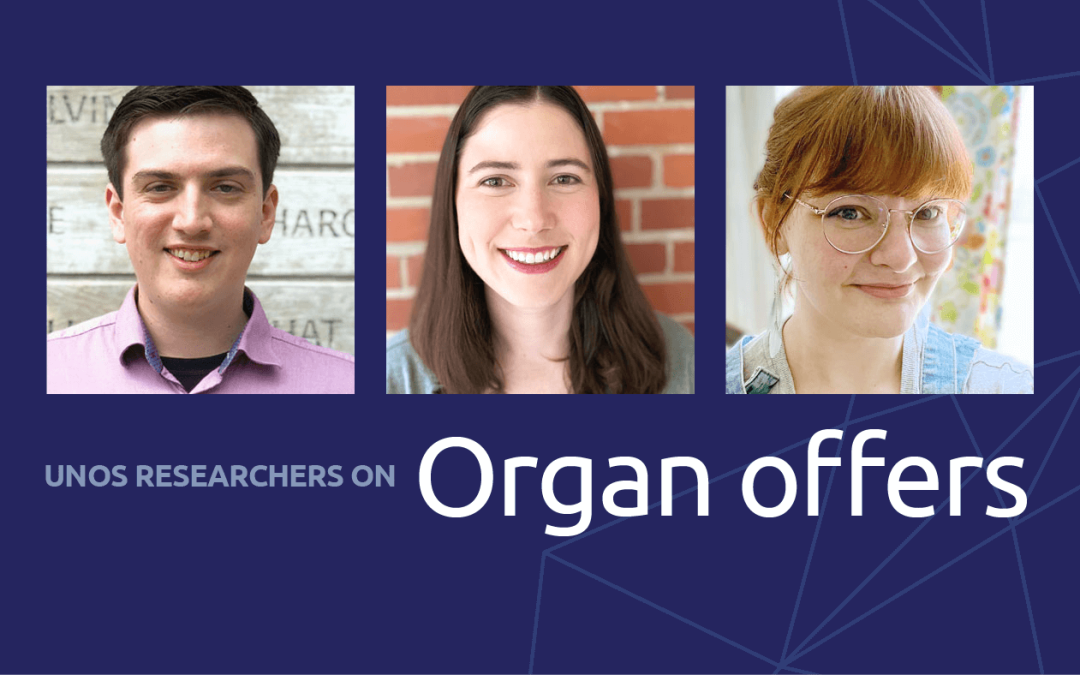
Research in focus: examining organ offers
Three recent studies from UNOS researchers examine offer acceptance practices and impact of Offer Filters tool.
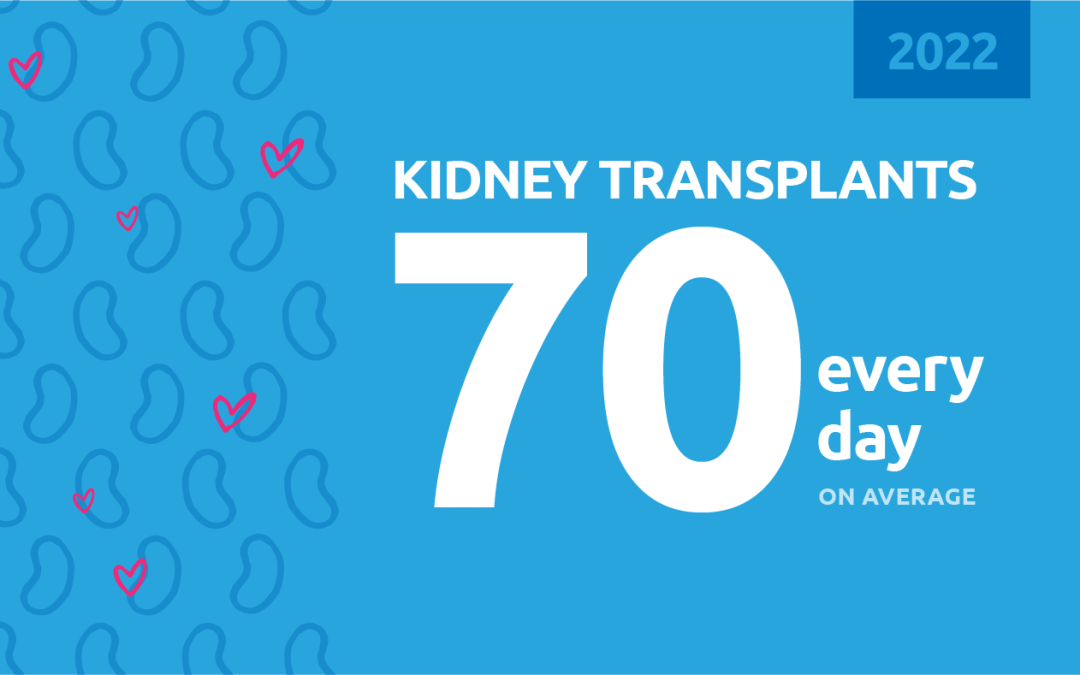
New milestone reached in kidney donation and transplant
For the first time, more than 25,000 kidney transplants were performed in a single year